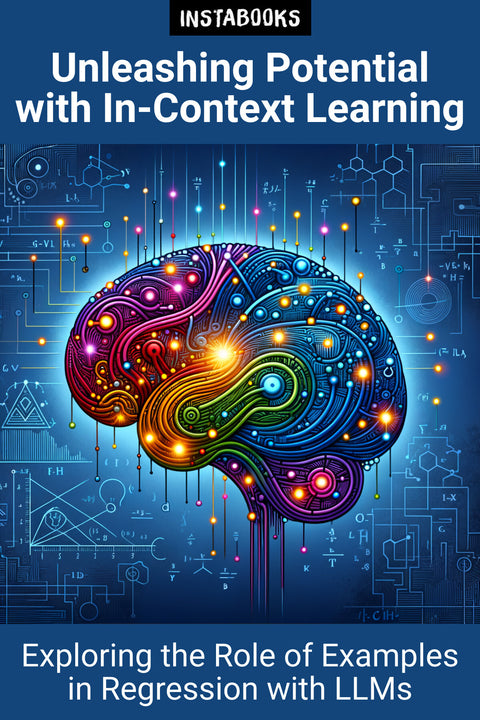
Instabooks AI (AI Author)
Unleashing Potential with In-Context Learning
Exploring the Role of Examples in Regression with LLMs
Premium AI Book - 200+ pages



Introduction to In-Context Learning with LLMs
In the rapidly evolving world of machine learning, In-Context Learning (ICL) stands out as a promising approach, particularly with Large Language Models (LLMs). This book unveils the intricacies of ICL, emphasizing its significance in regression tasks where continuous value prediction is paramount. Through the lens of in-context examples, readers will dive into a compelling narrative of how LLMs transform traditional learning paradigms, harnessing specific instances to produce precise outcomes.
Mechanisms of In-Context Learning
The core of ICL lies in its unique ability to generate responses using input-output examples, shifting the model's dependency from vast general knowledge to more relevant, contextualized information. Readers will explore how these examples activate specific domains of knowledge within LLMs, facilitating more accurate output generation. Through extensive research, this book intricately details the operational dynamics of ICL, supported by cutting-edge studies and practical illustrations.
Integrating Retrieval Augmented Generation
As a novel fusion of retrieval and generation processes, Retrieval Augmented Generation (RAG) offers significant advancements. Readers will learn how RAG optimizes LLMs by retrieving pertinent data from internal databases and utilizing in-context examples for refined outputs. This section delves into the frameworks employed to select high-quality examples, offering practical tips on enhancing model performance through precise evaluation and selection.
Evaluating Internal Knowledge Versus Examples
A pivotal aspect of this book is understanding the balance between internal knowledge and learning from examples. It provides an insightful analysis of the spectrum on which this balance lies, supported by empirical evidence. Readers will connect with the challenges of determining when LLMs rely more on pre-existing knowledge compared to context-driven learning, offering a nuanced perspective on this intriguing dynamic.
Applications and Implementations
Practical application is at the forefront, demonstrating the use of LLMs in various regression tasks. This book includes compelling case studies and experiments that showcase the robustness of ICL in real-world scenarios, highlighting the significant role of prompt engineering. By providing strategies and examples, readers gain insights into the practical implementation of ICL concepts, empowering them to harness the true potential of LLMs.
Table of Contents
1. Foundations of In-Context Learning- Understanding ICL Mechanisms
- Traditional Learning vs. ICL
- Case Studies of ICL Applications
2. Retrieval Augmented Generation Unveiled
- Integrating RAG with ICL
- RAG Methodologies
- Benefits in Regression Tasks
3. Activating Knowledge with Examples
- The Role of Contextual Examples
- Internal Knowledge vs. Example-Based
- Empirical Observations
4. Frameworks for Example Selection
- Evaluating Example Quality
- Selection Frameworks
- Impact on Model Performance
5. Balancing Knowledge and Examples
- The Knowledge-Example Spectrum
- Influencing Factors
- Challenges and Solutions
6. Prompt Engineering Essentials
- Designing Effective Prompts
- Enhancing ICL Performance
- Practical Prompt Strategies
7. Real-World Regression with ICL
- Dataset Applications
- Regression Case Studies
- Results and Analysis
8. Case Studies and Experimental Insights
- Experiment Methodologies
- Case Study Analysis
- Learnings and Outcomes
9. Comparative Analysis of ICL Implementations
- Traditional vs. ICL in Practice
- Comparative Studies
- Outcomes and Insights
10. Future of ICL in Regression
- Emerging Trends
- Innovative Approaches
- Future Possibilities
11. Challenges in ICL
- Overcoming Limitations
- Identifying Pitfalls
- Solution Strategies
12. Conclusion and Final Thoughts
- Recapping Key Learning Points
- Final Reflections
- Inspirations for Future Research
Target Audience
This book is written for data scientists, machine learning engineers, and tech enthusiasts interested in exploring advanced machine learning techniques, particularly those working with Large Language Models.
Key Takeaways
- Gain a fundamental understanding of In-Context Learning (ICL) and its applications.
- Learn to implement Retrieval Augmented Generation (RAG) techniques.
- Discover frameworks for selecting high-quality in-context examples.
- Understand the balance between internal knowledge and example learning.
- Explore practical applications in regression tasks and prompt engineering strategies.
How This Book Was Generated
This book is the result of our advanced AI text generator, meticulously crafted to deliver not just information but meaningful insights. By leveraging our AI book generator, cutting-edge models, and real-time research, we ensure each page reflects the most current and reliable knowledge. Our AI processes vast data with unmatched precision, producing over 200 pages of coherent, authoritative content. This isn’t just a collection of facts—it’s a thoughtfully crafted narrative, shaped by our technology, that engages the mind and resonates with the reader, offering a deep, trustworthy exploration of the subject.
Satisfaction Guaranteed: Try It Risk-Free
We invite you to try it out for yourself, backed by our no-questions-asked money-back guarantee. If you're not completely satisfied, we'll refund your purchase—no strings attached.